Researchers from DeepMind have developed a multimodal neural network capable of performing various types of tasks. For example, she can control a robot, play games for Atari, write text and describe photos. The article about the algorithm is published on arXiv.org, the authors also talked about it on the DeepMind website.
In 2017, researchers from Google Brain presented the neural network architecture Transformer, a distinctive feature of which was the widespread use of the attention mechanism. This allows the neural network to understand the context of words and sentences much better, which in turn has made great progress in the field of natural language processing in general. One of the most famous examples of this progress is the GPT-3 model from OpenAI. It turned out that if you train a model on a huge array of texts, it will learn a good representation of the language and how texts should look, after which it can be quickly and on a very small amount of data to train it to a specific task. Moreover, this task does not have to be textual: it turned out that GPT-3 can perform basic arithmetic operations.
In parallel with the development of universal language models, researchers are developing multimodal models that work simultaneously with different data. Researchers from DeepMind, led by Nando de Freita, have developed a new multimodal neural network Gato, which allows you to use the Transformer architecture to solve a variety of tasks.
Since Transformer was developed for language tasks, this architecture works with text tokens. Accordingly, to work with different data, Gato turns them into tokens. The developers used four tokenization schemes. The text is tokenized in a standard way, in which subwords are allocated in words and encoded with a number from 0 to 32 thousand. The images are divided into squares (16 by 16 squares), and the pixels in them are encoded from -1 to 1, and then these squares are fed into the model line by line. Discrete values are transformed into numbers from 0 to 1024, and continuous values are sampled and transformed into a number or a set of numbers from 32000 to 33024. If necessary, tokens can also be split by dividing tokens.
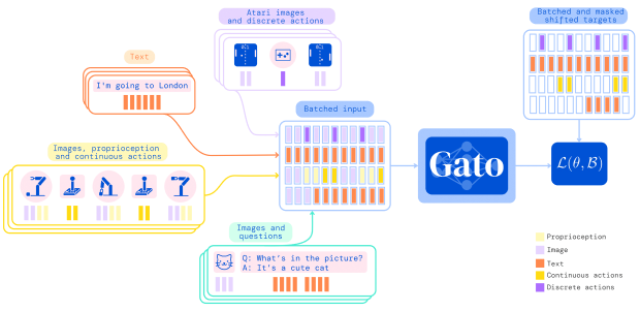
How the model works with different data
Image source: Scott Reed et al. / arXiv.org, 2022
After tokenization of incoming data, each token turns into embedding (in fact, a compressed vector representation of the same data) in two ways: for images, squares are passed through a convolutional neural network of the ResNet type, and for the rest of the data they are selected through a learned search table (since any token is an integer in a limited range).
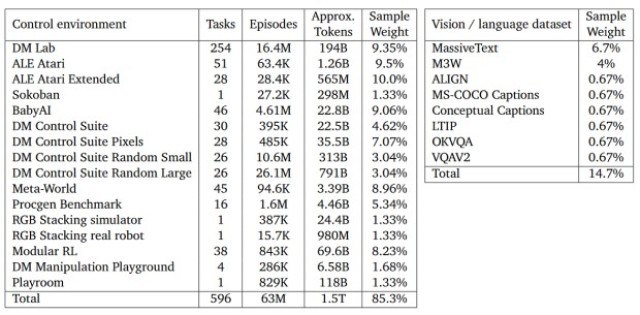
Datasets used for training
Image source: Scott Reed et al. / arXiv.org, 2022
The researchers used 24 datasets with different types of data and with their help trained the model to perform 604 tasks. At the same time, the model did not achieve record results on these tasks. In some, for example, in 23 games for Atari, it copes better than people, but this is not a new result for machine learning algorithms — in 2020, DeepMind developed an algorithm that beats people in 57 games at once. In others, it clearly does not reach the level of a person, for example, in the annotation of images:
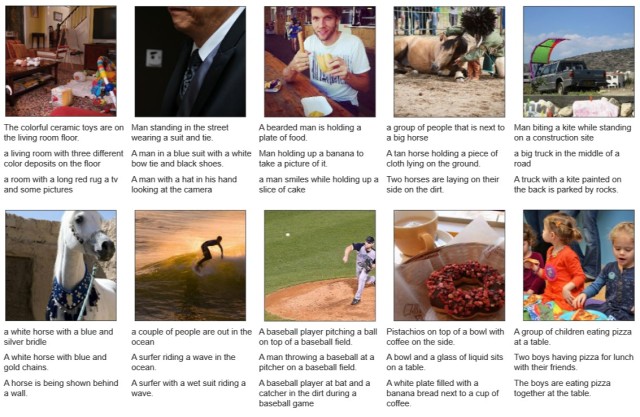
Examples of neural network image descriptions
Image source: Scott Reed et al. / arXiv.org, 2022
In fact, DeepMind demonstrated the opposite approach: instead of creating a highly specialized model that solves a specific task or a set of related tasks better than others, the developers created a universal model that solves the most tasks, but not very qualitatively.
In addition to multimodal neural networks, researchers are also working on multimodal methods of their training, that is, a single method suitable for training specialized models for working with text, images or sound. Recently we talked about such a method developed by developers from Meta.
Grigory Kopiev