A group of researchers from the Institute of Fundamental Sciences (IBS), Jens University and the Max Planck Institute have developed a new artificial intelligence (AI) technology that brings machine vision closer to how the human brain processes images. This method, called Lp-Convolution, improves the accuracy and efficiency of image recognition systems, while reducing the computational burden on existing AI models.
The human brain is surprisingly effective at recognizing key details in complex scenes, an ability that traditional artificial intelligence systems have difficulty reproducing. Convolutional neural networks (CNNs), the most widely used artificial intelligence model for image recognition, process images using small square filters. While effective, this rigid approach limits their ability to identify broader patterns in fragmented data.
Recently, Vision Transformers (ViT) has demonstrated superior performance when analyzing entire images in one go, but they require high computing power and large datasets, making them unsuitable for many real-world applications.
Inspired by how the visual cortex of the brain selectively processes information through cyclical, sparse connections, the research team tried to find a middle ground: could such an approach make convolutional neural networks both efficient and powerful?
To answer this question, the team developed Lp-Convolution, a new method that uses multidimensional generalized normal distribution (MPND) to dynamically shape CNN filters. Unlike traditional CNNs that use fixed square filters, Lp-Convolution allows AI models to adapt the shape of the filters — stretching them horizontally or vertically depending on the task, similar to how the human brain selectively focuses on important details.
This breakthrough solves a long-standing problem in AI research known as the big core problem. Simply increasing the size of filters in convolutional neural networks (for example, using 7×7 cores or more) usually does not improve performance, despite adding more parameters. Lp-convolution overcomes this limitation by introducing flexible, biologically based connection schemes.
In tests on standard image classification datasets (CIFAR-100, TinyImageNet), Lp-convolution significantly improved the accuracy of both classical models such as AlexNet and modern architectures such as RepLKNet. The method also proved to be very resistant to corrupted data, which is a serious problem in real-world AI applications.
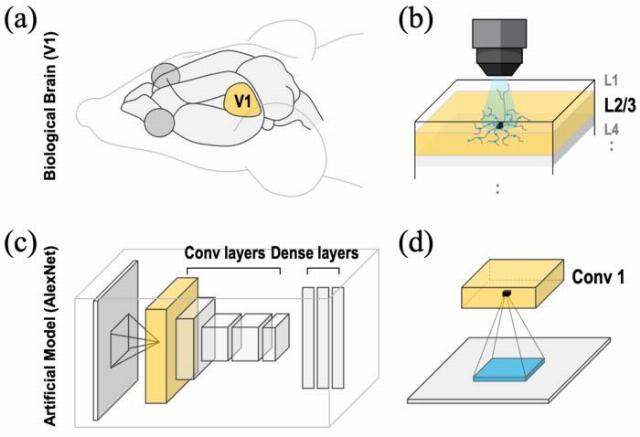
Image: https://www.semiconductor-digest.com Moreover, the researchers found that when the Lp masks used in their method resembled a Gaussian distribution, the AI's internal data processing exactly corresponded to biological neural activity, which was confirmed by comparison with data on the brains of mice.
"We humans quickly identify what's important in a crowded scene,— said Dr. Justin Lee, director of the Center for Cognitive and Social Research at the Institute of Basic Sciences. "Our Lp convolution mimics this ability, allowing AI to flexibly focus on the most important parts of an image, just like the brain does."
Unlike previous developments that either relied on small, rigid filters or required resource-intensive converters, Lp-convolution offers a practical and efficient alternative. This innovation has the potential to revolutionize areas such as:
– Autonomous driving, in which artificial intelligence must quickly detect obstacles in real time.
– Medical imaging, which improves artificial intelligence-based diagnostics by highlighting fine details
– Robotics that provides more intelligent and adaptable machine vision in changing environments
"This work is an important contribution to both artificial intelligence and neuroscience," said Director K. Justin Lee. "By linking artificial intelligence more closely to the brain, we have unlocked the new potential of convolutional neural networks, making them smarter, adaptable, and biologically realistic."